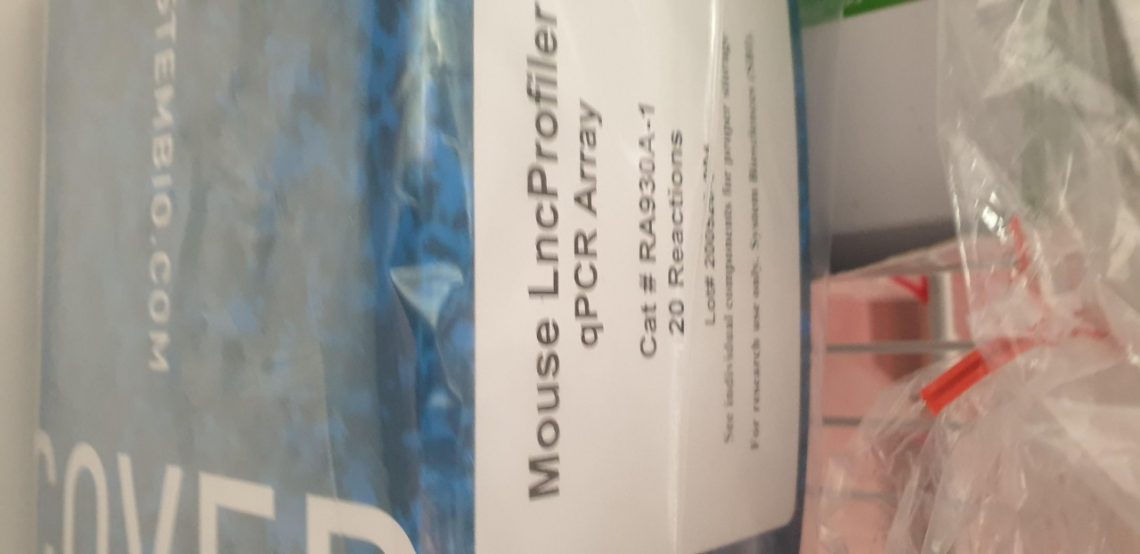
Robust Data Integration Method for Classification of Biomedical Data
Robust Data Integration Method for Classification of Biomedical Data
We present a protocol for integrating two types of biological data – clinical and molecular – for more effective classification of patients with cancer. The proposed approach is a hybrid between early and late data integration strategy. In this hybrid protocol, the set of informative clinical features is extended by the classification results based on molecular data sets. The results are then treated as new synthetic variables. The hybrid protocol was applied to METABRIC breast cancer samples and TCGA urothelial bladder carcinoma samples. Various data types were used for clinical endpoint prediction: clinical data, gene expression, somatic copy number aberrations, RNA-Seq, methylation, and reverse phase protein array.
The performance of the hybrid data integration was evaluated with a repeated cross validation procedure and compared with other methods of data integration: early integration and late integration via super learning. The hybrid method gave similar results to those obtained by the best of the tested variants of super learning. What is more, the hybrid method allowed for further sensitivity analysis and recursive feature elimination, which led to compact predictive models for cancer clinical endpoints.
For breast cancer, the final model consists of eight clinical variables and two synthetic features obtained from molecular data. For urothelial bladder carcinoma, only two clinical features and one synthetic variable were necessary to build the best predictive model. We have shown that the inclusion of the synthetic variables based on the RNA expression levels and copy number alterations can lead to improved quality of prognostic tests. Thus, it should be considered for inclusion in wider medical practice.
A Bacteria-Inspired Morphology Genetic Biomedical Material: Self-Propelled Artificial Microbots for Metastatic Triple Negative Breast Cancer Treatment
Morphology genetic biomedical materials (MGBMs), referring to fabricating materials by learning from the genetic morphologies and strategies of natural species, hold great potential for biomedical applications. Inspired by the cargo-carrying-bacterial therapy (microbots) for cancer treatment, a MGBM (artificial microbots, AMBs) was constructed. Rather than the inherent bacterial properties (cancerous chemotaxis, tumor invasion, cytotoxicity), AMBs also possessed ingenious nitric oxide (NO) generation strategy.
Mimicking the bacterial construction, the hyaluronic acid (HA) polysaccharide was induced as a coating capsule of AMBs to achieve long circulation in blood and specific tissue preference (tumor tropism). Covered under the capsule-like polysaccharide was the combinatorial agent, the self-assembly constructed by the amphiphilic dendrons with abundant l-arginine residues peripherally (as endogenous NO donor) and hydrophobic chemotherapeutic drugs at the core stacking on the surface of SWNTs (the photothermal agent) for a robust chemo-photothermal therapy (chemo-PTT) and the elicited immune therapy.
Subsequently, the classic inducible nitric oxide synthase (iNOS) pathway aroused by immune response was revolutionarily utilized to oxidize the l-arginine substrates for NO production, the process for which could also be promoted by the high reactive oxygen species level generated by chemo-PTT. The NO generated by AMBs was intended to regulate vasodilation and cause a dramatic invasion (as the microbots) to disperse the therapeutic agents throughout the solid tumor for a much more enhanced curative effect, which we defined as “self-propulsion”. The self-propelled AMBs exhibiting impressive primary tumor ablation, as well as the distant metastasis regression to conquer the metastatic triple negative breast cancer, provided pioneering potential therapeutic opportunities, and enlightened broad prospects in biomedical application.
Robust Data Integration Method for Classification of Biomedical Data
We present a protocol for integrating two types of biological data – clinical and molecular – for more effective classification of patients with cancer. The proposed approach is a hybrid between early and late data integration strategy. In this hybrid protocol, the set of informative clinical features is extended by the classification results based on molecular data sets. The results are then treated as new synthetic variables. The hybrid protocol was applied to METABRIC breast cancer samples and TCGA urothelial bladder carcinoma samples.
Various data types were used for clinical endpoint prediction: clinical data, gene expression, somatic copy number aberrations, RNA-Seq, methylation, and reverse phase protein array. The performance of the hybrid data integration was evaluated with a repeated cross validation procedure and compared with other methods of data integration: early integration and late integration via super learning. The hybrid method gave similar results to those obtained by the best of the tested variants of super learning. What is more, the hybrid method allowed for further sensitivity analysis and recursive feature elimination, which led to compact predictive models for cancer clinical endpoints.
For breast cancer, the final model consists of eight clinical variables and two synthetic features obtained from molecular data. For urothelial bladder carcinoma, only two clinical features and one synthetic variable were necessary to build the best predictive model. We have shown that the inclusion of the synthetic variables based on the RNA expression levels and copy number alterations can lead to improved quality of prognostic tests. Thus, it should be considered for inclusion in wider medical practice.
[Linking template=”default” type=”products” search=”rahul” header=”3″ limit=”166″ start=”4″ showCatalogNumber=”true” showSize=”true” showSupplier=”true” showPrice=”true” showDescription=”true” showAdditionalInformation=”true” showImage=”true” showSchemaMarkup=”true” imageWidth=”” imageHeight=””]
Aidi injection, a traditional Chinese biomedical preparation, for gynecologic tumors: A systematic review and PRISMA-compliant meta-analysis
Aidi injection (ADI), a traditional Chinese biomedical preparation, is a promising adjuvant therapy for gynecologic tumors (GTs), including cervical cancer (CC), endometrial cancer (EC), and ovarian cancer (OC). Although studies have reported positively on ADI therapy, its exact effects and safety in GT patients remain controversial. Therefore, a wide-ranging systematic search of electronic databases was performed for this meta-analysis. Data from 38 trials including 3,309 GT patients were analyzed. The results indicated that the combination of conventional treatment and ADI markedly improved the patients’ overall response rate (P < 0.00001), disease control rate (P < 0.00001), and quality of life (P < 0.05) compared with conventional treatment alone. Furthermore, patient immunity was enhanced with combined treatment, as indicated by significantly increased percentages of CD3+ (P = 0.005) and CD4+ (P < 0.00001) and increased CD4+/CD8+ ratio (P = 0.001). Most of the adverse events caused by radiochemotherapy such as gastrointestinal issues, leukopenia, thrombocytopenia, and hepatotoxicity, (P < 0.05 for all) were significantly alleviated when ADI was used in the GT patients. However, other adverse events such as nephrotoxicity, diarrhea, alopecia, and neurotoxicity did not significantly differ between the two groups. Overall, these results suggest that the combination of conventional and ADI treatment is more effective than conventional treatment alone.

